top of page
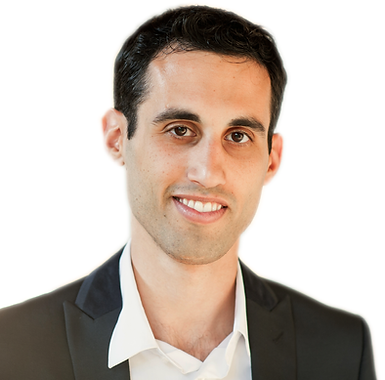
I'm interested in
Deep Reinforcement Learning
Representation Learning
Partial Observability & Causal Inference
A Recent Achievement:
I've completed a 5 year project (solo) - a 3d puzzle game for mobile - Barrel Cats.
Game is currently being reviewed by several mobile publishers.
Check out the promo video
Partial Observability: Discovering and exploiting the causal structure in an environment is a crucial challenge for intelligent agents. It allows for transfer of knowledge to new unfamiliar situations, as well as the ability to make unbiased decisions when parts of the environment are unobserved or unknown, yet still affect our observations and decisions.
Learning Representation: To be successful in real-world tasks, reinforcement learning needs to exploit the compositional, relational, and hierarchical structure of the world and transfer it to the task at hand. It is vital to learn abstractions that allows us to generalize, communicate plans, intentions, and requirements for efficiently solving the problem at hand.
bottom of page